Probability & Partners: Establishing a climate stress testing framework in banks
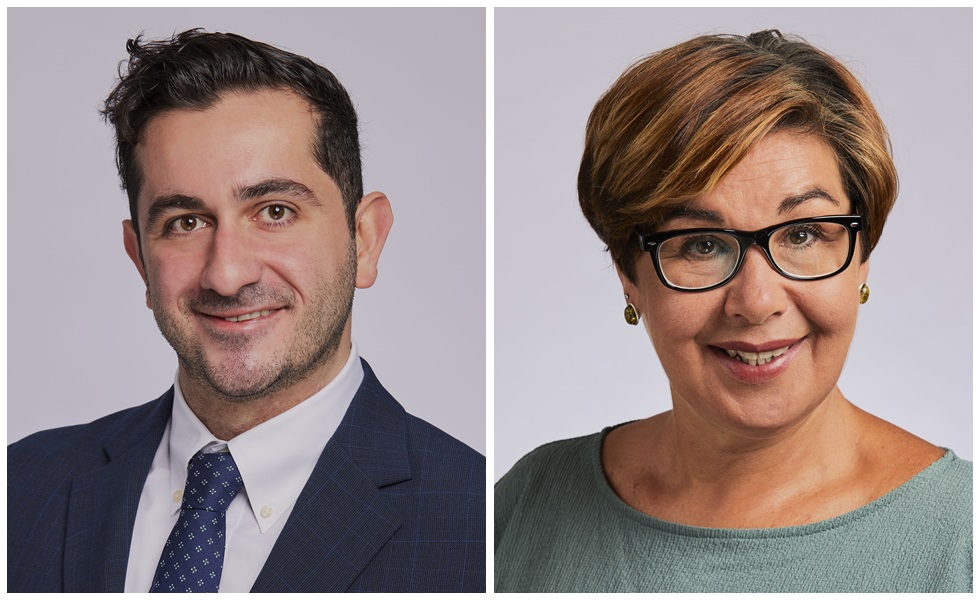
By Kamuran Emre Erkan, Quantitative Consultant, and Svetlana Borovkova, Head of Quant Modelling, both at Probability & Partners
At Probability & Partners, we have recently started developing a climate risk stress testing framework for banks.
With the focus on mortgage portfolios, we also implemented the framework for sectoral portfolios with distinct collaterals, like data centres or moveable assets. The data, scenarios and the methodology to build the framework thus needed to be different for every portfolio. What were some of the main data issues and methodological challenges we encountered during this initiative?
Data issues
A primary issue we encountered was sourcing publicly available data from entities like Klimaateffectatlas (for physical risk maps), Startanalyse (for transition costs) and NGFS (for emissions, electricity prices and the electricity use amounts).
One important problem was the underestimation of the required time to collect the data. Most of the publicly available data is collected through contacting the data providers via email: that response time might take longer than expected.
Another problem was understanding the definitions and the structure of data. For example, the tools to access or view the data or the details (columns or the description of entry points) of the data are not completely clear. This translates to additional time, to read and comprehend.
In other words, publicly available data doesn’t imply a trivial process. It is important to put emphasis on this in the planning and scheduling of the project.
Another issue we encountered during the data preparation phase was matching data from various sources. This was the most time-consuming step of the whole project. Matching data is not a trivial task and it can get computationally expensive as well, which makes the whole process even more challenging. Matching physical risk maps with loan data was particularly challenging due to the non-standard nature to use geographical data in quantitative finance roles. Thus, it requires an additional effort and a learning curve.
An additional challenge was matching data from different departments within banks. It is possible that collected ESG-related data is differently structure than the loan specific data, and it might get challenging to match these two data sets without a clear description of the data tables that are available. Therefore, it is good practice to have clear descriptions of the available data to ease this process.
Methodological challenges
In our methodology, we concentrated on costs emerging from physical and transition risks of the scenarios. However, due to the lack of available data and limited scenario-specific literature, we had to rely on average costs derived from other studies. This raises the question whether to employ more granular cost data than available averages. The decision requires careful consideration of available data, collateral, and specific scenario factors.
If it is decided to get data that is more granular than average, then the next step is to decide in what dimension to get more granularity. In our approach, we saw that bucketed average costs were more effective for transition risks, whereas for physical risk it proved that scaling from the average cost (or another reference point like maximum cost) was beneficial.
It is also important to monitor how these buckets and scaling are impacting the stress on the portfolio, to make sure the costs are not unreasonably underestimated or exaggerated. This might end up with recurrent data preprocessing with new proxies for the missing data.
Another challenge is to translate these costs into capital calculations, which mostly translates as measuring the impact of these costs on Probability of Default (PD), Loss Given Default (LGD) and Exposure at Default (EAD). In literature this is typically done by translating these costs into financial indicators like loan to value ratio (LTV), return on equity (ROE), debt service coverage ratio (DSCR), et cetera. However, because this step is highly dependent on the assumptions about how climate stress costs are going to be translated into these metrics, it requires a comparison of several assumptions.
The next step is to translate these financial indicators into PD and LGD, while EAD is mostly dependent on whether or not there is an additional borrowing. The challenge therefore is to build a relationship between the financial indicators and PD/LGD. This is a sector/portfolio specific relationship, possibly with limited literature, and it also requires an answer to the question: do we look for a fundamental relationship, or a relationship based on a bank’s internal loan data?
Thorough preparation and monitoring
In conclusion, we can say that our experience showed us that for climate risk stress testing there is a considerable challenge at virtually every stage. It is essential to make a realistic plan and schedule beforehand to allow these challenges enough time and effort. Also, due to the lack of available data and knowledge on the topic, proxies and assumptions potentially influence the outcomes of the stress testing. It is important to monitor and test these assumptions and be aware of the weaknesses of the framework, in order to have a robust understanding of the results.
We think that the climate stress testing approach currently being invented for banks is also valuable for pension funds, insurance companies and asset managers.